A Grid Refinement Study of Alg-PC
We conduct a grid refinement study for the algorithm using piecewise constant model on the second example in paper. $\mathcal{G}$'s are chosen as $N\times N$ uniform square decompositions, with $N = 10, 20, 40$. We use $W_*$ instead of the learnable optimality $\tilde{W}_*$ (see here) to compute the regret metrics of performance.
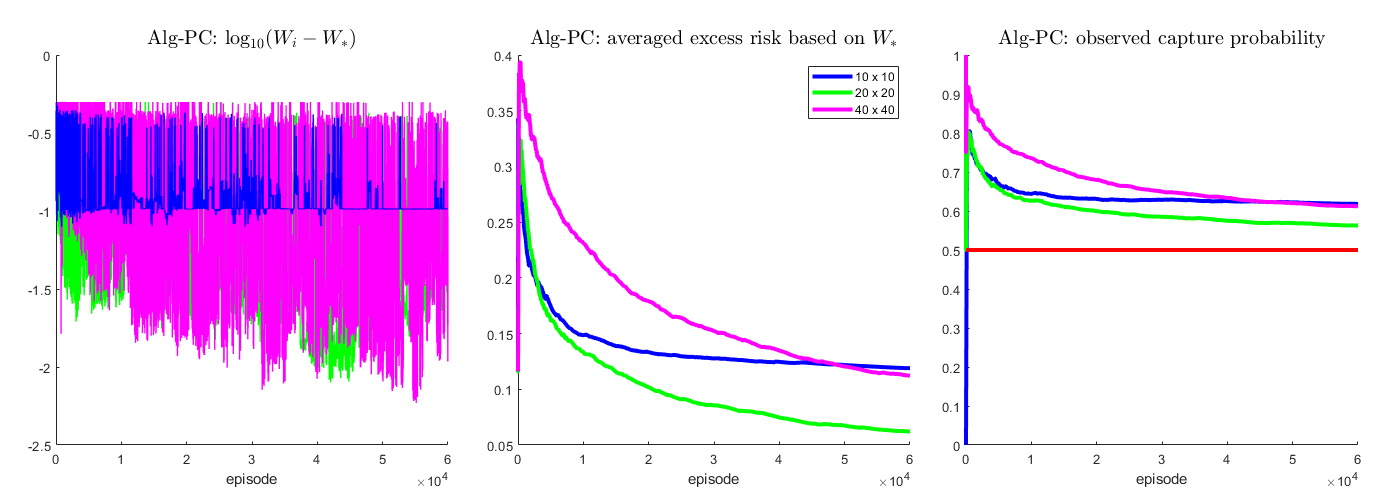
As we can see from the middle figure, with $T = 60,000$ episodes, Alg-PC yields a lower averaged regret on a 10$\times$10 $\mathcal{G}$ than on a 40$\times$40 $\mathcal{G}$ for the first 50,000 episodes while the averaged regret on a 20$\times$20 grid (can be viewed as a compromise between a quite fine grid with too many cells and a quite coarse grid with much fewer cells) is much smaller and decays faster throughout. The right figure consolidates this through another regret metric, the experimentally observed capture probability.
In the left figure for $N = 20$ and $N = 40$, we observe that the (non-averaged) differences between $W_i$ and $W_*$ are pushed down to a much lower level, compared with $N = 10$. This indicates that with $N = 20$ and $N = 40$ Alg-PC is able to explore paths which are closer to become optimal.